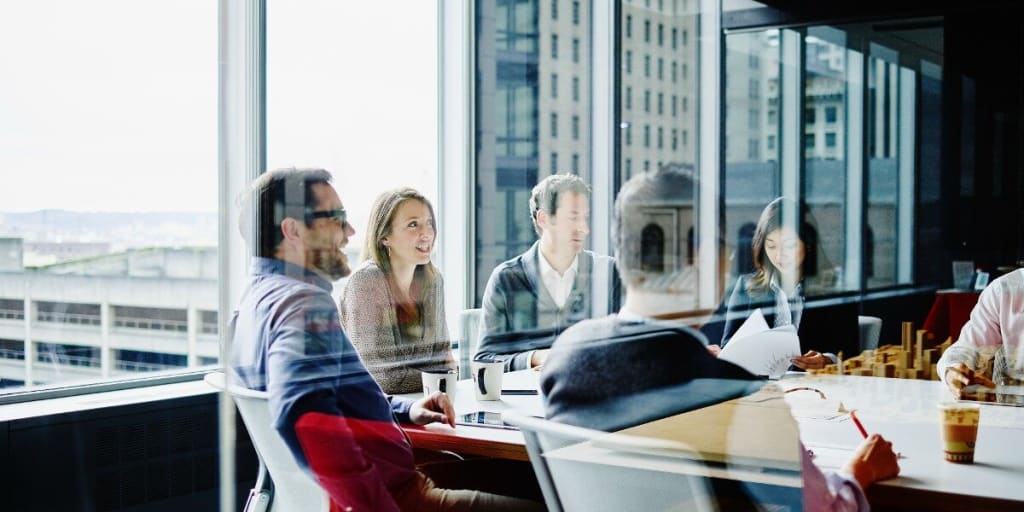
From descriptive to prescriptive: CRE managers need to overhaul their portfolio analytics
Heads of corporate real estate (CRE) have known for a long time that they need to improve how they analyze usage of their portfolio resources. As it becomes increasingly obvious that hybrid work is here to stay, the implications of this on the size, distribution, and character of portfolios are enormous. But making changes to any of these three factors, as well as others, will be costly. Now more than ever, CRE executives need reliable and comprehensive data to spot a need for change—and then justify any transformative decisions they subsequently make. The risk of getting it wrong is fundamentally driving investments into next-generation portfolio analytics.
Off the back of any analytics, there is wide range of potential paths. These can involve consolidating or expanding the portfolio, exiting a property, renewing a lease contract, selling a building, sub-leasing or even redesigning a floor, and relocating to new markets.
JLL Technologies (JLLT) identifies five levels of analytics that can be used to inform decision-making:
- The what: Descriptive, fact-based analyses, which focus on a situation or event. This is something most CRE organizations already do, such as describing the number of sites that are leased or the total square footage of a portfolio.
- The how: Augmented analyses, which involve layering descriptive analytics with additional data sets. This is done to answer more complex questions. Examples may include how occupants use different types of space or how rent compares to the local market average.
- The why: Diagnostic assessments, which use trend comparisons and correlations between different metrics to help identify possible causal factors behind trends in the portfolio. This is often done to establish a baseline or norms around attendance rates, or to explain unusual events, such as a spike in operational costs or high occupancy.
- The when: Predictive estimates, which are predicated on forecasting when events may occur, such as occupancy spikes, and are mostly based on historical data. This is done to inform planning efforts and give operational teams an idea of the minimal resources they may need to safely accommodate staff needs.
- The which: Prescriptive analyses, which are based on generated scenarios of resource allocation and determine which scenario could deliver the best outcome. This is done to guide planning and operational actions, such as selecting the right amount of equipment for a given site to provide the most optimal workplace for the occupants most likely to turn up.
Analytics in practice: What CRE executives get wrong about return-to-office initiatives
Corporate enterprises are now on a hybrid continuum. Some, such as Netflix, Snapchat, Tesla, and Twitter, are office-centric, with employees expected to be in the office all week. Others are fully remote, such as Dropbox, Reddit, and Slack. But the majority are somewhere in the middle. Think Google, Meta, Microsoft, or Salesforce. For most firms, they have some form of initiative to push more staff back to the office at least a few days per week. The aim of this is to encourage collaboration and foster meaningful workplace culture, with its associated benefits on productivity and talent management.
But, for this to happen, the CRE team needs a firm handle on the following (at the very least):
- The supply of space
- The true level of demand
One of the most significant challenges JLLT sees with many companies is that their headcount forecasts do not provide information about location, roles, alternative working locations, or staff schedules. If you cannot anticipate any of these, it will be challenging to know if a return-to-office initiative has any chance of improving collaboration and culture. Merely knowing the total attendance rate, for instance, and seeing that is it climbing over time, could you give a false sense of hope that the CRE function is genuinely delivering value to the organization.
Analytics are only as strong as the information put in: Critical data elements determine reliability
Critical data elements (CDEs) are pieces of discrete information that are necessary to define, measure, and compare occupant demand as well as supply. They carry specific formats to ensure that you can rely on them for consistent usage and calculations. To create occupancy zones (or neighborhoods), for example, pieces of data from multiple systems must be classified in a consistent manner and bounded into a single location, including area names, space measurements, seating, and amenities. And for the return to the office, zoning helps define the kind of work you expect to be done in particular areas.
Again, this is an example of how good analytics underpin measurements of how successful return-to-office initiatives truly are. If you wish for the finance department to work more with the CRE department on capital project planning, in the same area, for instance, you need to get your CDEs in order to facilitate this. Coordinating this cross-team collaboration and measuring specific space usage over time requires CDEs.
Proven practices point the way to next steps
Below are some proven practices JLLT has observed with its own client base:
- Direct staff time toward establishing a master list of properties. This means a dedicated system of record, with standardized naming and coding for all properties, to serve as a foundation block for broader analytics (including AI-driven analyses). Such a list can be independent of an IWMS platform, which is often used for leasing or space booking, and a CMMS solution used for maintenance management. Once the list is established, you can more easily see multiple levels of detail at aggregate, regional, or market level, in addition to by property type, to name a few examples.
- Recruit help from the entire organization. As the workplace now covers areas beyond the physical office, CRE teams must work in closer collaboration with HR (e.g., headcount), IT (e.g., work equipment details), finance (e.g., cost profiles), procurement (e.g., supplier information), and product delivery (e.g., role details). This will inevitably involve collecting data from or even integrating with non-CRE enterprise IT systems to facilitate more comprehensive workplace analytics.
- Set data quality goals and reach them. Fundamentally, this comes down to documenting the logic and structure behind your data sets to institutionalize the knowledge needed to understand as well as update them. This enables apples-to-apples analyses and boosts the data literacy of your CRE team.