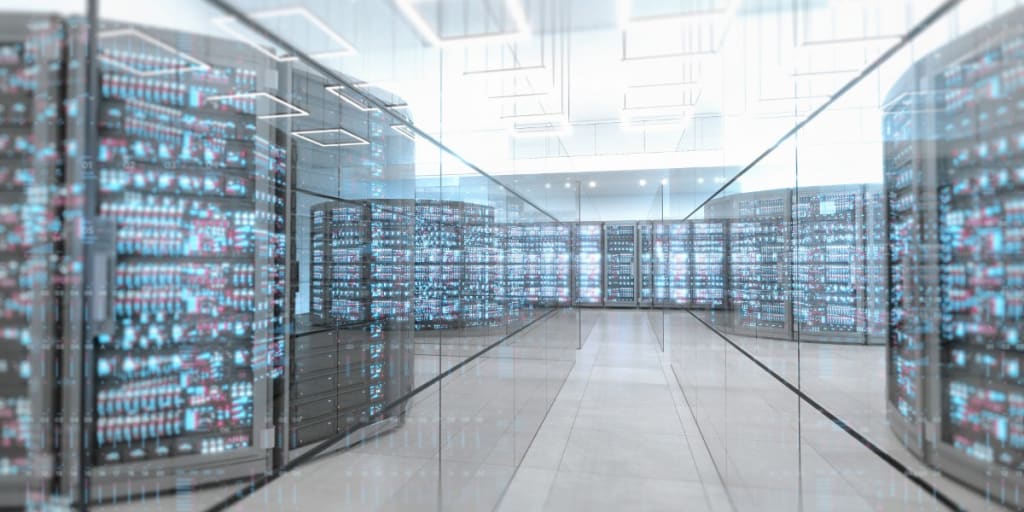
The impact of AI on CRE
Imagine if you started your day tomorrow, and Microsoft Excel disappeared overnight. Gone. Never existed. Most of the world would simply grind to a halt. Much maligned, but obviously necessary, spreadsheet apps are the fabric of modern knowledge work.
AI (artificial intelligence) apps are the next Excel. In a not so far future, we’ll certainly be integrating them into our daily work.
ChatGPT has become the shiny new object of AI apps. It’s a no-code, easily accessible use of AI, with a tremendous number of use cases, many of which create an existential disruption to the modern knowledge worker. Cue the rapidly spreading mantra, “ChatGPT won’t take your job, but someone who knows how to use it will.”
Commercial real estate (CRE), like any other industry, must embrace this rapidly evolving technology. AI is no longer an abstract, esoteric technology used by data scientists or programmers. It is now available to almost any knowledge worker. It’s easy to get lost in the storm of excitement and disruption. While ChatGPT is getting a lot of attention, it’s just one kind of use case for AI. When it comes to CRE solutions, we need to be aware and clear about all kinds of use cases for AI and distinguish which AI is the best kind to solve different kinds of problems.
Despite the exciting advances in AI, CRE problems have remained relatively unchanged. For occupiers, operating cost management remains critical, and portfolio optimization remains elusive. Occupancy demand is uncertain as companies tune their return-to-office programs. And for both occupiers and owners, transaction volume has continued to lag compared to prior years, making exits and disposals highly tricky. To manage through these challenges, CRE must be able to identify and manage the drivers of success: financial discipline, operational excellence, superior occupant experience, and continuous learning.
AI offers potentially new ways to do this. But with all new capabilities and opportunities, there’s a learning curve—and with it comes the potential for mistakes. CRE leaders need to be able to define, sponsor, and support the development of Applied AI: picking the right tool for the right problem.
What is AI, anyway? McKinsey generally defines it as “a machine’s ability to perform the cognitive functions we associate with human minds, such as perceiving, reasoning, learning, interacting with an environment, problem-solving, and even exercising creativity.” That can dive very deeply into the particulars of AI methodologies, including machine learning and deep learning. There are plenty of good overviews for any of us to read further. But let’s bring that down to simpler, practical definitions. We can refer to it as Applied AI, where can AI be used to solve problems, in particular, CRE problems.
We can generalize Applied AI into three different applications and uses: generative, predictive, and optimizing:
As you can see, ChatGPT-like Applied AI is only one of several uses of AI for CRE, which is why it’s so important for real estate leaders to be fluent in the use cases. We don’t want to incur technical debt, especially when we don’t have a good grasp on the problem statement and the appropriate technology to solve it!
It’s relatively early in the Applied AI journey to say how much is at stake, but we have unfortunate history to teach us. Various organizations track estimates of the cost of the wrong technology choice every year, both in terms of explicit costs to remediate it as well as the lost GDP as a result of it. Depending on what’s included and what’s not, estimates range from $50-100 billion a year in terms of pure remediation to well over several hundred billion dollars, accounting for the opportunity cost of wasted GDP.
On a brighter note, what can we do be successful with Applied AI?
Go for big data
Provide data—and lots of it. No matter the use case, AI involves training and learning. The more data that we can provide the Applied AI, the greater the experience of the tool. Applied AI needs big data: massive bodies of data that can be evaluated for relatedness and representing human or natural behaviors that have traditionally been difficult to predict using traditional rules and logic. An important question to ask: What is the amount of data needed to actually help the AI work and learn? For CRE, good examples include the engineering experience of buildings or the market dynamics experiences for the prices of asset classes.
Prepare for training and supervision
Greater experience does not necessarily mean greater success. We have the example of Microsoft’s Tay, which was meant to learn from Twitter conversations to generate “casual and playful conversation.” Within 24 hours, Tay had not only absorbed a tremendous amount of content, but it began to engage in a way that it learned would generate the greatest amount of “signal.” Unfortunately, this content was far from casual or playful. This spectacular failure underlined the importance of continuing guidance and training.
Set realistic expectations
At least for now, Applied AI will be used to enhance our productivity or potentially accelerate our ability to make decisions. It does not, however, replace human oversight and final decision-making. The limitations of experiential data and the ongoing requirements for supervision cannot allow us to simply let them run by themselves. But it does require changes in how we operate—specifically, additional training for knowledge workers. Knowledge workers will have to interpret the initial suggestions from the Applied AI, determine final input or decisions, and provide feedback to the Applied AI on the quality of its suggestion.
That’s a challenging set of things to do, and it might even seem a bit daunting. Where might you begin? Not all CRE organizations may have the existing skills to build or acquire the Applied AI or the budget to acquire the kind of data that might be needed.
Make the business case for the investment
It helps to start by defining your business case. Where might you be spending considerable time on backlog or remediation activities that are creating waste and inefficiency, such as:
- Manually reconciling or matching like information. For example, multiple instances of IWMS or CMMS systems.
- Sense-making and classification of information. For example, the abstraction of leases.
Or, perhaps, complex problem-solving that takes longer than you have time to decide, such as:
- Occupancy stacking, where you are trying to co-locate related functions and business processes for a minimum of space.
- Making building engineering decisions with imperfect information concerning expected occupancy, weather patterns, or equipment performance.
A business case will consider the explicit or opportunity cost of the time and effort needed to solve these problems. How much do these processes cost, in terms of people, time, and money? What kinds of things could they be doing rather than these backlog or remediation efforts? Could they, for example, spend time actually fixing the redundancies that drive reconciliations? Negotiating better outcomes with vendors and lessors? These can be presented against the investments in Applied AI for longer-term ROI.
Expect doubts and resistance to change
Realize that there’s going to be valid insecurity and concerns about Applied AI. It will, inevitably, displace knowledge workers from the kind of work that can be accelerated and even improved by Applied AI. There’s also valid concerns about the quality and reliability of what Applied AI can do. Applied AI, left unsupervised or managed, can rapidly evolve toward generally incorrect outcomes, or may, on rare but significant exceptions, provide specific but completely incorrect conclusions. These represent significant reputational, operational, and financial risks to your organization, your customers, and your stakeholders. Often, doubts and resistance are healthy. When harnessed into good oversight, quality management, and teamwork, they can provide excellent governance.
Seek opportunities for teamwork and collaboration
When it comes to harnessing doubts and resistance to change for better outcomes, it’s all about teamwork and collaboration. Applied AI isn’t simply data science and programming. You’ll need a wide set of contributors, starting with those who can help frame the business problem and make sure that you’ve clearly defined your use cases. You’ll need to team up with:
- Those who currently do the work that you are seeking to augment (most important!): There’s no one who better knows the complications and nuances of the existing work. And, if you are going be successful, they will be the ones supervising and training it.
- Owners of data: Acquiring and wrangling data isn’t always easy, and you’ll need the time investment from the owners of big data coming from CRE engineering, facilities maintenance, or occupant interaction.
- Technology management: It will be a tricky, somewhat disruptive shift for your technology organization to properly engage in Applied AI; table-based data run by technology for basic workflow or simple analytics won’t be enough. You’ll need higher speed ingestion of data, deeper computing capabilities, and more innovative ways of structuring the data.
- Others working with Applied AI: You’ll want to engage those who are just getting started or already working with Applied AI. With new and rapidly evolving technology, you’ll need a community that can share key learnings and insights.